“[A]ny State claiming a pioneer role in the development of new technologies bears special responsibility for striking the right balance in this regard” – The European Court of Human Rights in the S. and Marper v. the UK case (4 December 2008) [para. 112]
1 Introduction
Fundamental taxpayers’ rights impose on regalian authorities the obligation to be in capacity to interpret the decisions of AI and machine-learning (ML) models. This cornerstone obligation can be derived from several fundamental rights, e.g. the right to a private life, right to a fair trial, or right to non-discrimination. Whether used as a mean of assistance or as an agent of the tax administration, these fundamental rights explicitly preclude the existence of technical or legal black-boxes, which effectively would render these rights entirely moot. Cases such as SyRI, eKasa, the toeslagenaffaire and Ligue des droits humains strongly reinforce the notion that transparency and explainability are conditio sine qua non to the use of AI as a method of public governance, including tax administration. Yet, however obvious, this postulate is met with enormous resistance whereby public and private authorities attempt to uphold barriers – non-natural born killers – to delay the inevitable. In the US, the killer of XAI is embodied by trade secrecy and the protection of intellectual property of the developer of AI models, as seen in COMPAS. Property rights are upheld as superior to digital constitutionalism and defendants’ fundamental rights. In the EU, tax administrations promote the notion that institutional secrecy is indispensable to prevent tax crimes, which trumps taxpayers’ fundamental rights. Despite different killers, the result is the same, the integration of technology to public governance seriously erodes fundamental rights. In this second post, we propose an alternative balancing equation, a roadmap towards transparency and explainability through a blend of legislative and technical measures that accounts for the interests of the (tax) administration and the administered (taxpayers). The overarching goal is to achieve a symbiotic relation between these actors, not the Pyrrhic victory of one.
2 The roadmap to ‘holistic explainability’
Prior to balancing interests of the tax administration and the taxpayers, it is important to acknowledge the sort of pressure tax officials face, with an ever-increasing administrative burden, in part resulting from the succession of additional taxation rules, such as DACs, GAARs, Country-by-Country Reports. Tax administrations have to process countless more volumes of data, with substantially fewer human resources. Yearly, each tax administration processes billions of returns, spends millions of minutes on the phone and answers millions of e-mails. In such a context, technology is not only an ally of choice, but a pre-requisite to the performance of their missions. Yet, fundamental rights may not become moot by virtue of a software upgrade. The use of technological tools by tax administrations should be anecdotal and seamless to taxpayers’ rights. Receiving an appropriate explanation from the tax authorities whenever they rely on AI systems would contribute to the taxpayer’s trust in the functioning of such systems. It would also be informative for judges when they resolve disputes between stakeholders that use AI systems and the ‘victims’ of the lack of their explainability. In the long run, disputes of that nature will decrease because the number of disputes in that sphere is inversely proportional to the AI systems’ degree of explainability. For that to happen a proactive approach of regulators across the world need to be taken.[1] To strike the right balance between the values and rights of tax administrations or developers of AI systems protected by tax and trade secrecy, on the one hand, and those of taxpayers, on the other, a new set of rules and practices must be developed. The analysis in Part I identified at least two distinct categories of fiscal institutional secrecy, depending on the moments where secrecy is invoked. These distinct moments obey to different predicaments which trigger various public interests to balance. The question of how to achieve these balances, of where to place the cursors, ultimately depends on the moment in time where the need for explainability arises.
Interestingly enough, transparency and explainability are often characterised in literature as independent requirements. Yet, in the context of fiscal institutional secrecy, transparency and explainability serve the same cause – ensuring that taxpayers are informed. So much so can be seen in eKasa, where the safeguards prescribed by the Slovak Constitutional Court are: transparency, auditing, and explainability, all measures combatting secrecy. The Slovak court identifies the timeline and interplay between the principles: transparency is prophylactic while explainability serves as the cure. These two principles are communicating vessels that form part of the same system of ‘holistic explainability’. Conceptualizing transparency and explainability as a system enables a more agile and conciliant approach, where the lack of transparency may be compensated by additional explainability in court, and vice versa. Opaque legislation and State practices spills over to the judicial system by increasing the demand for explainability. Initially transparent legislation would bear the opposite effect. In fine, it is for policy-makers to decide which trade-off they prefer.
2.1 Measures for legislative transparency
To uphold legislative transparency, models posing a risk of conflict with taxpayers’ rights should be regulated through legislation. Prior studies have shown how in the EU, only a small minority of Member States using AI systems for tax enforcement have adopted a specific norm to that effect. AI systems used by tax administrations have transformed tax enforcement practices to such an extent that in many aspects these do not correspond to procedures reflected in tax codes. Most tax procedural codes in the EU do not mention the use of statistics as a method of selection of taxpayers or the use of web-scraping, some pre-date the use of the internet or the telephone. The distribution of power and constitutional balance has changed. The famous ‘information asymmetry’ in disfavour of the administration appears more and more as a distant myth. These systems simply cannot be regulated through vestigial tax procedure, but should be subject to novel legislative norms. It constitutes the sole channel where the balance between the use of these systems and the limits to interferences with taxpayers’ privacy, data protection and non-discrimination can be legitimately discussed assented. The limits of web-scraping and the sources of data collection for tax enforcement, the meanings of fairness and discrimination in taxation, the options to object automated decisions should all be normatively framed via specific regulations. Algorithmic good governance simply cannot rest on outdated legislation.
The law should prescribe a clear, concise and accurate description of the different tools used by their respective tax administration and an inventory of the categories of data processed. In that regard, Article 4-1 of the French CFVR regulation should be highlighted as a positive example of algorithmic governance. The CFVR regulation cites the different sources of data, both internal and external, including governmental databases, judicial and municipal records and the different websites from which taxpayer data is extracted. In addition, the regulation provides an accurate description of a number of AI tax enforcement systems leveraged by the French tax administration. Similarly, the Belgian 2012 regulation cites a number of AI tools used by their tax administration, and their underlying purposes. These two norms do not provide a complete inventory of all the tools leveraged, but comparatively to the vast majority of EU Member States, these represent a clear step in the right direction. Within the EU, these two States are pioneers in the use of AI tax enforcement systems, and are currently the most transparent about such use. These norms are testimony to the fact that tax enforcement will not be jeopardized by legislative transparency. Norms regulating AI tax systems should also provide taxpayers sufficient verifiable insights on how the risks of conflict with taxpayers’ rights will be neutralised by the administration. Currently this requirement is lax throughout the EU. A simple illustration of this is the fact that the terms ‘bias’, ‘statistical bias’ or ‘discrimination’ are entirely absent from norms meant to regulate AI tax systems. Given how prevalent the risk of bias is in the context of AI, and how prominently it is cited, such an absence speaks volumes. These norms should advise on standards of statistical and algorithmic performance, data pre-processing, calibration, parity, etc. Again, by virtue of the use statistics for audit and risk-scoring, the very essence of fairness and non-discrimination can be impacted. Algorithmic good governance should contain measures to show how such impact will be managed.
2.2 Measures for judicial explainability
Regarding judicial explainability, if AI systems are used in the decision-making process of the tax administration, as a mean of assistance or for the pre-selection of taxpayers, these systems should enable intuitive interpretation. Institutional secrecy cannot serve as an excuse to deprive taxpayers of their right to a motivated decision. Methods exist to ensure explainability while maintaining the black box. This can be done through human interpretation, if the model is intuitively explainable. Risk-scoring systems of the tax administration often are relatively simple to interpret, with a limited number of variables that can be understood by a human agent. For more complex AI systems, counterfactual models the likes of LIME or SHAP may be used, although their suitability to given stakeholders require further research. A balanced approach should be able to differentiate between the information received by the court and the taxpayer. The former might receive more granular information, including the data used to train the AI model and the weight assigned to each statistical risk-indicator. These are the bare essentials to determine the output that led to the decision of tax authorities in the taxpayer’s case to enable a guided assessment of the system’s performance. In the case of COMPAS, access to the features of the model was denied to the defendant. Faúndez-Ugalde et al. report that in Chile, taxpayers’ right to access is protected and taxpayers can receive some information on the models used, e.g. metrics of performance and accuracy. Judges could be equipped with specific application programming interface (API) with tools to operate a thorough algorithm audit, for instance FairTest kits. The information obtained by taxpayers does not need to be quite granular. Instead, the taxpayer might receive, upon request, an explanation from the tax authority detailing the decision and why it took precedence over plausible alternatives. This differentiation in the scope and content of the explanation of AI systems is justified by the obligation of the court to deliver a judgment backed up by reasonable and persuasive arguments in support thereof. Taxpayers bear no such burden. For them, it is sufficient to understand why the AI system led to outcome X rather than outcome Y (counterfactual) and that such an outcome is or is not predicated on unfair or discriminatory elements.
Some argue that even with such tools, judges may lack the necessary expertise to operate algorithm audit – ‘who watches the watchers’ criticism. Judges are typically not trained in machine learning and statistics, judex non calculat, hence cannot determine whether the counterfactual explanation is correct. Despite being highly accurate, counterfactual models are also prone to some errors themselves. Using a model to explain another model presupposes expertise over two machine learning systems and impartiality in their evaluation. Yet, in the same length, judges cannot sequence DNA, perform an autopsy or analyse fingerprints but these sorts of evidence are regularly admitted in courts. If judges lack the expertise to operate counterfactual models, they are in capacity to appoint independent experts to do so. The lack of expertise of the judge does not constitute a barrier to many forensics tests, and should not be with AI tools either.
3 Conclusion
Fiscal institutional secrecy is often pictured as an axiom of tax enforcement. Yet the examples of France and Belgium suggest the opposite, that legislative transparency bears no effect on the capability of the administration to enforce taxation rules. The same can be said of judicial explainability. As aptly observed by the European Convention on Human Rights in S. and Marper v. the UK of 4 December 2008 in the case concerning the retention of DNA profiles for an indefinite term, “any State claiming a pioneer role in the development of new technologies bears special responsibility for striking the right balance in this regard”. This observation is very much valid nowadays in respect of the use of AI by tax administration and in other spheres of public powers.
Simple cost-effective technological explainable solutions shall be developed to ensure that taxpayers receive an appropriately motivated decision for their grievances and for judges to accurately audit AI tax systems. In the negative, public actors such as tax administrations should opt for intuitively interpretable models, that can be explained by a human agent or else refrain from using statistics to aid decision-making. In fine explainability and transparency should be viewed on the same spectrum. Transparency and explainability form communicating vessels, whereby the lack of one will impact other regalian institutions. The impact of initially opaque legislation will be felt on the judicial system by increasing the demand for a motivated explanation. Transparent norms could alleviate that pressure. It is for policy-makers to decide whether this pressure is sustainable to their governance model. Holistic explainability may only be achieved via a blend of measures, ensuring that taxpayers understand algorithmic outcomes at every moment identified on the timeline.
Ultimately, courts and legislatures should wonder whether tax secrecy is the hill to die on, when studies show that open governance and cooperation are catalysts for tax compliance. Respect for taxpayers’ constitutional rights increases tax morale, induces compliance and bears a dampening effect on tax evasion. Institutional secrecy is a façade only. Seasoned experts can and have deduced what may lead to an audit from the administration, the red flags that will trigger a tax official. In reality institutional secrecy only punishes disfavoured taxpayers without sufficient means to afford these experts. Standards on transparent and explainable AI would only contribute to a more equal distribution of that knowledge.
[1] As early as in 2017, Elon Musk urged U.S. Governors to regulate AI: “AI is a rare case where I think we need to be proactive in regulation instead of reactive. Because I think by the time we are reactive in AI regulation, it’s too late.”
Błażej Kuźniacki, Research Associate at the Centre for AI and Data Governance (CAIDG) – the Singapore Management University; Associate Professor at the Lazarski University; Advisor at the PwC Global Tax Policy Team and & Senior Manager at the International Tax Services Business Unit, PwC Netherlands
David R. G. Hadwick, Doctoral researcher in Tax & Technology at the DigiTax Centre of Excellence, University of Antwerp; PhD Fellow at the FWO – Research Foundation for Flanders, Belgium
________________________
To make sure you do not miss out on regular updates from the Kluwer International Tax Blog, please subscribe here.
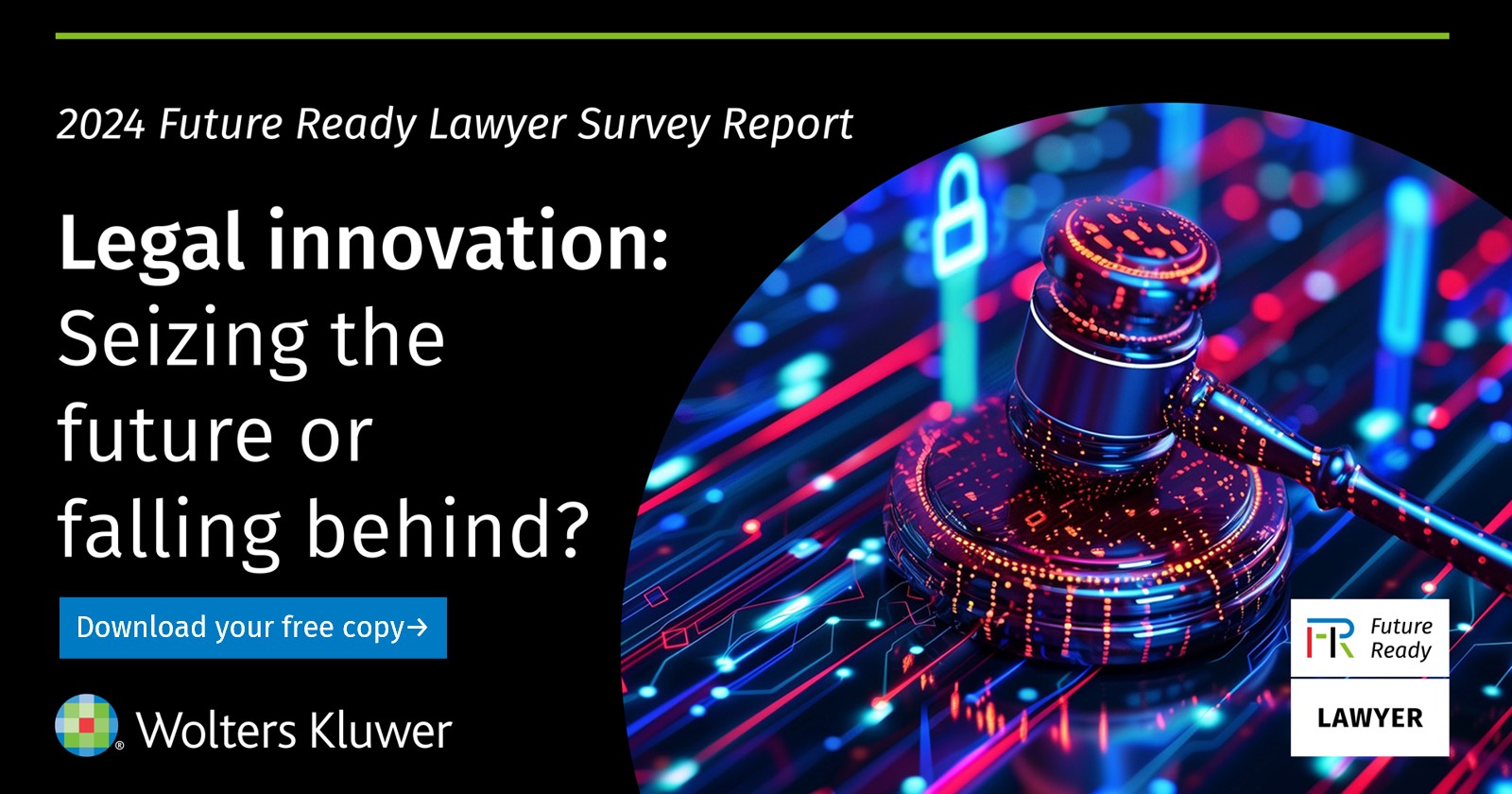
