“[I]n this world, with great power comes great responsibility!” – Uncle Ben advice to the young Peter Parker, the 1962 Amazing Fantasy #15, by Stan Lee
1 Introduction
Explainable artificial intelligence (XAI) systems in tax law are needed to comply with principles of law such as legality, proportionality and non-discrimination. A sufficient degree of explainability is also indispensable to fundamental taxpayer’s rights, for example the right to respect for private and family life. Currently, the obstacles to XAI may not be related to models’ technological complexities and sophistications, such as deep neural networks, but predominantly stem from a decision of the judiciary or legislature. The major to XAI is secrecy, the non-natural (because purely legal) born killer of XAI in tax law. In the US, secrecy seem to mostly take the shape of trade secrecy, while in the EU it is first and foremost institutional. In fine, the effects are the same – barring citizens, including taxpayers, access to information on algorithmic means of profiling. Given the emergence of more complex systems, the likes of generative AI and ChatGPT, the adoption of XAI standards should be reactive and pre-empt a spill over of less intuitively interpretable models to tax administrations’ processes. Particularly amidst the adoption of the OECD Pillar I and Pillar II as new standards to achieve fairness in taxation (however debatable), the argument should be raised that XAI equally contributes to fairness by upholding taxpayers’ fundamental rights (however overlooked by policy makers).[1]
In this first post, we compare the two types of secrecy and both continent’s approach to the right to access information on algorithms leveraged by the State. In the second part, we will try to indicate how to kill these killers by striking the balance between the need of tax and trade secrecy for tax authorities and tech companies, on the one side, and the need to understand how tax AI affects taxpayers, on the other.
Our underlying message is that states and multinational enterprises (MNEs) deploying AI around the globe shall not hide these powerful technologies behind tax and trade secrecy to diminish the responsibility for their potential negative consequences. Inevitably, “in this world, with great power comes great responsibility!”.
2 Trade secrecy
Trade secrecy in respect of AI plays a similar role to tax secrecy, i.e., preventing a discovery of the inner functioning of AI systems. There are legitimate reasons behind the drive to protect trade secrecy such as economic incentives, promoting innovation, protecting outcomes of long and costly R&D process. Even cybersecurity can sometimes validate the necessity to maintain certain practices or technology purposefully opaque, for instance to prevent adversarial attacks or theft. Considering that such models may have been developed by companies through many years of research in a specific field, a certain secrecy can be expected. Yet, similarly to tax secrecy, protection against reverse-engineering alone cannot justify the deprivation of transparency and explainability. An appropriate balance between different rights and values must be stricken down.
Trade secrecy constitutes a barrier to explainability, both in the private and in the public sector. Police, courts and tax administrations regularly collaborate with tech companies to license or build and deploy AI systems in public domains. In all such cases, the source codes of AI systems become highly protected trade secrets of tech companies. The way of functioning of algorithms in AI systems and even data used to train them may be proprietary. Accordingly, the use of proprietary models by public actors could lead to an erosion of standards of explainability and reasoned decisions. This can for instance be observed, in Wisconsin v. Loomis where the State Supreme Court found no violation of due process rights for litigant who was denied access to information about COMPAS, because Northpointe, the proprietor of the model invoked trade secrecy. This judgment was later confirmed by the Federal Supreme Court in State v Loomis. The defendant, Eric Loomis, argued that it was impossible to challenge a risk assessment without sufficient information about how AI system functions, e.g., how risks are determined and how factors are weighed to calculate the assessment. By contrast, the court stated that due process rights were not infringed because the defendant had access to the data used as an input to AI system in order to verify its accuracy.
The focus on data quality by the court was rightly criticized by scholars, since data quality standards only set a low bar to understand predictive risk assessments. Data science literature establishes that data accuracy is just one of many data qualities and does not fully address how the algorithm generates outputs or processes new input. A risk assessment algorithm processes data from a multitude of sources with ranging degrees of accuracy, labelling it, extracting variables and features, weighting inputs, and generates outputs all with an equally varied degree of biases and inaccuracies; all of these were ignored by the court in deciding against the right of defendant to get access to the mentioned information in order to ensure due process. By analogy, such an interpretative approach may be also followed by other courts in tax cases in which tax administrations use AI systems to score risk of tax frauds. Here, a double wall is built between the taxpayers and the explainability of AI systems: (1) trade secrecy (as in the Loomis case) and (2) tax secrecy (as in SyRI and eKasa cases).
3 Tax secrecy
Prior to operating a balancing exercise between the prerequisite to maintain secrecy for the administration and the taxpayers’ necessity to be informed about States’ institutional practices, one must understand what sort of tax secrecy is being developed in this post. Ontologically, the assessment of the origins of tax secrecy is rather complex, because unlike all other kinds of transparency, tax transparency does not refer to the disclosure of information by the State, but disclosure of information by taxpayers to the State, originally viewed as the short end of an information asymmetry. Tax secrecy is typically defined as the other end of that spectrum, that the information provided by a taxpayer is confidential to the taxpayer and the administration or other State’s organs. In other words, tax secrecy ensures that the information provided by taxpayers are only shared vertically, not horizontally to the public or other taxpayers. This post does not refer to this ‘tax secrecy’. Instead, it deals with the opacity of the tax administration regarding its institutional practices, including through the use of AI, including machine learning algorithms (sub-symbolic AI) and knowledge-based methods (symbolic AI), for the purpose of tax enforcement. This institutional secrecy rests solely on one question: whether the disclosure of institutional practices, or parts thereof, would jeopardize tax enforcement.
3.1 Tax secrecy and legislative transparency
Tax secrecy does not commence at trial, in fact litigation rather constitutes the very end of the spectrum of transparency. As per inter alia the constitutional principle of legality, taxpayers should already have been made aware of being subject to algorithmic decision-making through foreseeable, concise and transparent legislation. In the seminal works of Hobbes, Montesquieu, Kant, Bentham or de Tocqueville this is referred as ‘publicity’, i.e. providing citizens with sufficient information about an official activity. Transposed to AI algorithms, ‘publicity’ was rebranded by contemporary legal scholars (Pasquale; Citron; Hildebrandt) as ‘algorithmic transparency’, i.e. providing sufficient information on the use of AI systems by public regalian authorities (or private actors acting at their behest). The supply of information to taxpayers serve several purposes identified in literature and case-law, most notably: informational self-decision and accountability.
In the SyRI case of 5 February 2020, the Court of the Hague temporarily halted the use of Systeem Risico Indicatie, a machine-learning model of the Belastingdienst (the Dutch Tax and Customs Administration) meant to predict the risk of tax fraud. The plaintiffs argued that SyRI, as a predictive model used by a public authority, presented a significant risk of discrimination, accrued by the fact that the model had been tested on specific areas of the Netherlands, not necessarily representative of the entire Dutch population. The Court agreed with the plaintiffs and found that in light of these risks of discrimination, the legislation authorizing the use of SyRI did not provide taxpayers with sufficient verifiable insights on how these risks would be neutralized. The Court baptized this doctrine ‘transparency in the interest of verifiability’ (§6.91).
A similar logic can be seen in eKasa case of 17 December 2021 in which the Slovak Constitutional Court temporarily halted the use of a machine-learning system intended to process the data transferred by the electronic cash register system, mandatorily imposed on sellers. The Court found that while the mandatory use of the eKasa system was lawfully obliged by a legal basis, the processing of data on buyers and sellers through algorithmic means was not. The use of machine-learning algorithms was found incompatible with the principles of legality and transparency. As highlighted by the Court, the lack of a lawful base for the use of AI systems generates two prejudicial effects: first, on informational self-decision as it effectively bars taxpayers from knowing about the existence of the AI, and thus from self-assessing the potential impact, necessity and proportionality of such system; second, it absconds the public authority from any accountability regarding the oversight of taxpayers’ rights (§121). In the words of the Court: “The consequence of the application of technological progress in public administration cannot be an impersonal state whose decisions are inexplicable, unexamined and at the same time no one is responsible for them.”(§127) To negate the risks of algorithmic governance, the Court prescribes three safeguards: (i) transparency; (ii) collective supervision, in particular through audits from independent institutions, including civil society or academia; and, (iii) individual protection, for instance by providing access to the inner logic of the AI system, i.e. explainability (§132). Unlike the case of COMPAS examined in Loomis case, the Slovak Court foresees the application of these safeguards whether the issuer of the AI model is a private or public actors, superseding trade secrecy. It is noteworthy that the mentioned above three categories of safeguards relate not directly to the AI itself, but rather to tax secrecy, viewed by the Court as the real culprit of the case or the real externality to be dealt with.
The ruling in SyRI is a carbon copy of the judgment of the Slovak Court in eKasa. Both courts acknowledge the risks to taxpayers’ rights generated by the integration of AI systems. Yet both courts do not prescribe measures to directly negate the risks of discrimination, for instance through technical standards of fairness in machine-learning. Rather, these courts oblige legislatures to enhance the transparency of these processes, conscious that no current standard of computer science or statistic is able to deal with all externalities and outlier cases. In other words, even with best efforts errors are bound to happen and transparency is fundamental to deal with the aftermath of such errors. The black-box sub-symbolic AI is then viewed as the primary source of risks to taxpayers’ rights, by barring the possibility of identifying the nature of these errors.
The need for deployment of explainable AI systems was underlined not only by national courts but also by the supranational. The Court of Justice of the European Union (CJEU) stated in the Ligue des droits humains case of 21 June 2022 case, regarding the automated processing of passenger name records, that “given the opacity which characterises the way in which artificial intelligence technology works, it might be impossible to understand the reason why a given program arrived at a positive match. In those circumstances, use of such technology may deprive the data subjects also of their right to an effective judicial remedy enshrined in Article 47 of the Charter.” (§195).
The similarity of jurisprudential reasoning and outcomes in cases concerning the use of AI in public domain indicates that States’ postulates of viewing tax secrecy as an absolute necessity for tax enforcement, is fading. In both cases, tax administrations are pictured as a ‘white-knight’ requiring secrecy and the absence of public scrutiny to perform its missions, in the likes of a vigilante. In both cases, these arguments are quashed by the Courts, prescribing the very opposite as a necessary pre-condition for the use of AI algorithms by tax administrations. This indicates a slight shift of rhetoric and balance in favor of taxpayers and against institutional secrecy. These cases do not dismiss the necessity for a certain degree of institutional secrecy, but clearly preclude the existence of technical and legal black-boxes. Inevitably such onus requires in practice that the administration reveals some information about the inner logic of the model, whether that is specific risk-indicators, specific taxpayer data used for training or specific statistical techniques leveraged in the course of the development of the AI.
3.2 Tax secrecy and judicial explainability
The second moment where institutional secrecy may be invoked is in the context of a litigation between taxpayers and the administration. In that sphere, the necessity to maintain institutional secrecy about AI systems should be weighed against the right to a fair trial and good administration, particularly the rights of the plaintiffs to be arm in arm with the State. In practice, the scope of information to be produced by the tax administration is substantially narrower in comparison to legislative transparency. Plaintiffs in principle do not require information about the entire model, simply information about their individual decision and what factors led to that treatment. Due to the nature of objection procedures, taxpayers who complain are the ones who bring arguments forward, which must then be rebutted by the administration. In such cases, the administration must simply produce credible evidence demonstrating that the complaint is unfounded. In literature, counterfactual explanations are highlighted as an appropriate method to test these complaints. Allegations of discrimination are based on specific protected grounds which can be computed in the form of a ‘what if?’ test, akin to how counterfactual models operate. This type of explanation could be provided through human interpretation if the model is intuitively interpretable, or through models such as LIME or SHAP. Studies show that these models are not infallible, yet for tax administrations regularly leveraging machine-learning technology, such externalities should be a good starting point in ensuring some explainability of AI systems. In the negative, machine-learning should perhaps not be used at all, in the same way that a lab technician should not be using a lab absent the ability to deal with hazards. As ruled in SyRI and eKasa, the onus is on the administration to demonstrate ex-ante compliance of the use of AI systems with taxpayers’ rights, including compliance with the obligation to motivate decisions. The motivation without a sufficient explainability is not motivation.
4 Conclusion
Secrecy adopts different shapes depending on the continent where it is invoked and adjudicated. In the US, secrecy seems to primarily take the shape of trade secrecy, while in the EU secrecy is first and foremost institutional. Accordingly certain differences between the two jurisdictions can be observed. In eKasa, the Slovak Court ruled that the nature of the actor, public or private has no bearings on the necessity to maintain safeguards. To a certain extent this pre-emptively disavows the possibility to invoke trade secrecy in future litigations. EU courts, including the CJEU, by way of eKasa, SyRI and Ligue des droits humains cases seem to attach further importance to the risks of discrimination and automation bias comparatively to the US. In those cases, discrimination is acknowledged and even emphasized by the courts. As such, this may be due to the fact that Eric Loomis, tried to invoke gender-based discrimination, an exogenous factor hardly subject to debate in criminology. On the other hand, in Loomis, the US Court allowed the defendant to verify the data inputted to the system. Such a measure cannot be observed in European jurisprudence, where the primary objects of the litigation are legislations, not judicial decisions. In the EU pressure was exclusively exercised over legislatures, while in the US the judiciary was of prime concern. These different framings may explain the differences in the two continents.
[1] As a side note, it is worth to acknowledge that the OECD vigorously promotes the use of AI by tax administrations around the globe (see Tax Administration 3.0) but does not appear to be equally eager in promotion of legal provisions to ensure XAI in tax domain. Although the OECD Council on Artificial Intelligence emphasizes that AI actors should commit to transparency and responsible disclosure regarding AI systems (see here), deployments of AI by tax administration is not mentioned at all. Moreover, the recommendations of the OECD Council, as opposed to rules regulating tax and trade secrecy, are not legally binding. They are therefore likely to be ineffective until implemented as hard law, with the resultant lifting of secrecy for the benefit of explainability. We did not hear and could not find any documentation of the OECD that would encourage states to implement legally binding requirements by states to ensure XAI in tax law. Curiously, the OECD seems to not be very inclined in promotion of transparency also in other areas. In respect to arbitration under tax treaties, see an apt observation of Stefano Castagna here at p. 439: “Interestingly, while within the investment arbitration context there has been a push for transparency of the proceedings, it is the OECD itself that acknowledges that States may want all information provided to be kept private.” See the 2017 OECD Commentary to Article 25, para. 80.1.
Błażej Kuźniacki, Research Associate at the Centre for AI and Data Governance (CAIDG) – the Singapore Management University; Associate Professor at the Lazarski University; Advisor at the PwC Global Tax Policy Team and & Senior Manager at the International Tax Services Business Unit, PwC Netherlands
David R. G. Hadwick, Doctoral researcher in Tax & Technology at the DigiTax Centre of Excellence, University of Antwerp; PhD Fellow at the FWO – Research Foundation for Flanders, Belgium
________________________
To make sure you do not miss out on regular updates from the Kluwer International Tax Blog, please subscribe here.
Kluwer International Tax Law
The 2022 Future Ready Lawyer survey showed that 78% of lawyers think that the emphasis for 2023 needs to be on improved efficiency and productivity. Kluwer International Tax Law is an intuitive research platform for Tax Professionals leveraging Wolters Kluwer’s top international content and practical tools to provide answers. You can easily access the tool from every preferred location. Are you, as a Tax professional, ready for the future?
Learn how Kluwer International Tax Law can support you.
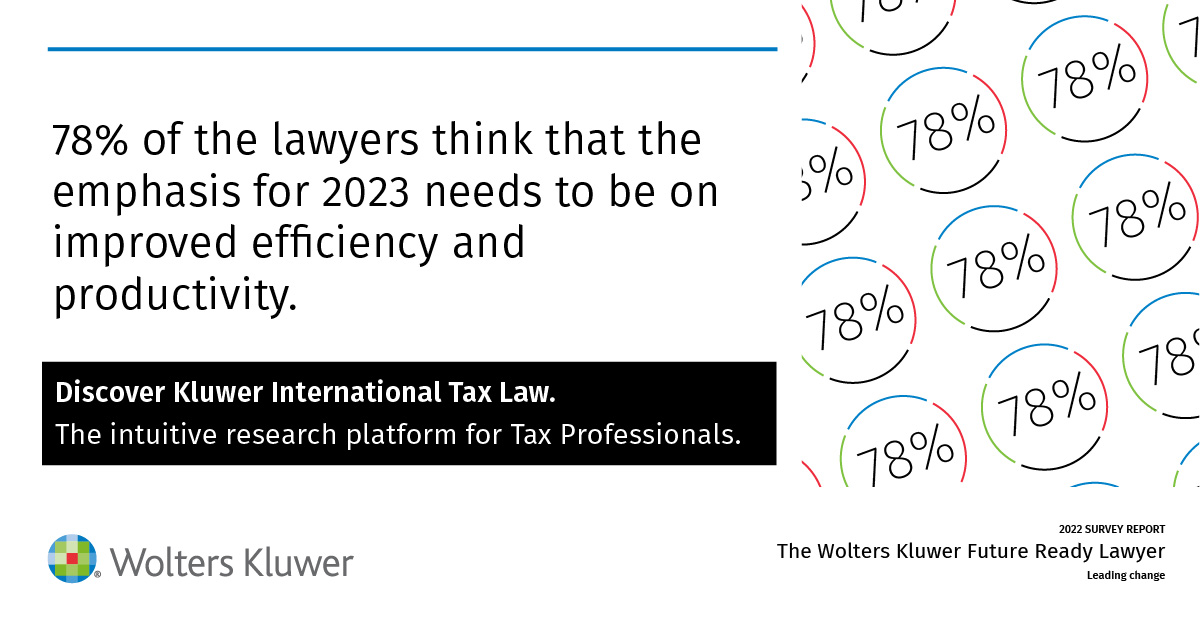
